Situated Language Understanding with Human-like and Visualization-Based Transparency
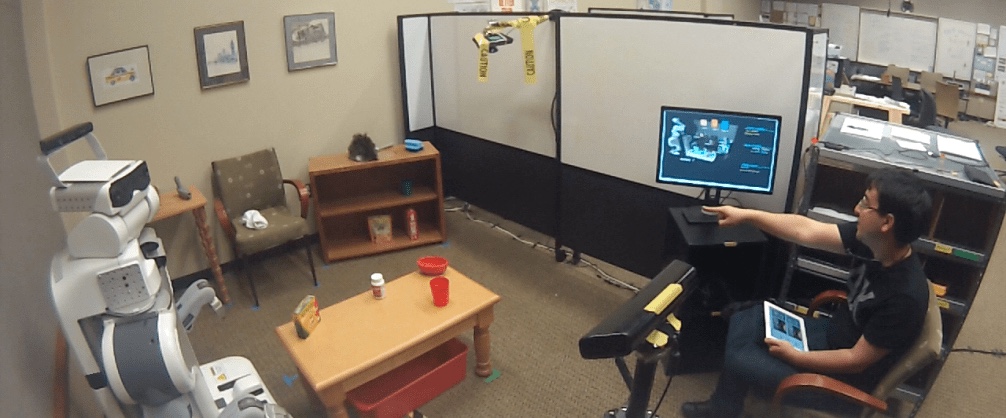
L. Perlmutter, E. Kernfeld, and M. Cakmak, “Situated Language Understanding with Human-like and Visualization-Based Transparency,” Robotics: Science and Systems (R:SS), 2016, doi: 10.15607/RSS.2016.XII.040.
Abstract
Communication with robots is challenging, partly due to their differences from humans and the consequent discrepancy in people’s mental model of what robots can see, hear, or understand. Transparency mechanisms aim to mitigate this challenge by providing users with information about the robot’s internal processes. While most research in human-robot interaction aim towards natural transparency using human-like verbal and non-verbal behaviors, our work advocates for the use of visualization-based transparency. In this paper, we first present an end-to-end system that infers task commands that refer to objects or surfaces in everyday human environments, using Bayesian inference to combine scene understanding, pointing detection, and speech recognition. We characterize capabilities of this system through systematic tests with a corpus collected from people (N=5). Then we design human-like and visualizationbased transparency mechanisms and evaluate them in a user study (N=20). The study demonstrates the effects of visualizations on the accuracy of people’s mental models, as well as their effectiveness and efficiency in communicating task commands.
BibTeX Entry
@article{perlmutter2016rss, title = {Situated Language Understanding with Human-like and Visualization-Based Transparency}, author = {Perlmutter, Leah and Kernfeld, Eric and Cakmak, Maya}, year = {2016}, booktitle = {Robotics: Science and Systems (R:SS)}, doi = {10.15607/RSS.2016.XII.040}, type = {conference}, url = {https://www.roboticsproceedings.org/rss12/p40.html}, video = {https://www.youtube.com/watch?v=Dj1_12PEkGo&t=13s} }